Hi, I’m Daan, an interdisciplinary master-student of urban and architectural history. These last few months, I worked as an intern for the Amsterdam Time Machine. As part of my internship, supervised by Melvin Wevers and Chiara Piccoli, I evaluated Google’s new tool Rǝ. It’s pronounced ‘Return’, but we usually refer to it as ‘Re.City’. One might call it Google Maps with a historical dimension. While we focus on Amsterdam, Google aims to make a Time Machine for the entire world. Through crowdsourcing, Google seeks to gather metadata, maps, pictures, and even 3D models of historical cities. In their 3D platform, Re.City will generate all buildings existing in a chosen timeframe as basic meshes created from their building footprint and their associated height information. Using a time slider, users can travel in the 2D or 3D environment from 1800 to 2000. At first glance, this fits perfectly with the mission of the Time Machine project, and the Amsterdam Time Machine in particular. For this reason, my internship aimed to go through the whole pipeline of Re.City to evaluate the platform and identify which input data are needed.
To avoid constructing 3D models of all historical buildings in Amsterdam anew, I first gathered existing datasets containing relevant metadata, maps, and 3D models. Recent LIDAR-scans could also be used since many of the existing buildings in the city center are in fact historical buildings. To assess whether they existed in the past, we also gathered metadata on the construction and, in some cases, the demolition date. However, we could not upload all this information in bulk and assign the correct metadata to the buildings. This requires manual labor on our end, and the data needs to be accompanied by specific attributes that are not included in, for instance, the 3D BAG dataset by the TU Delft.
Furthermore, we extracted the building information from Adamlink. By doing this, we immediately obtained a list of buildings with construction dates that we could add to the Re.City environment. It was possible to add these building footprints and associated height information in bulk. However, this task was not so straightforward when we tried to upload the footprints of more detailed 3D models from 3D BAG. They consist of segmented footprints, each segment being associated with a different height value.
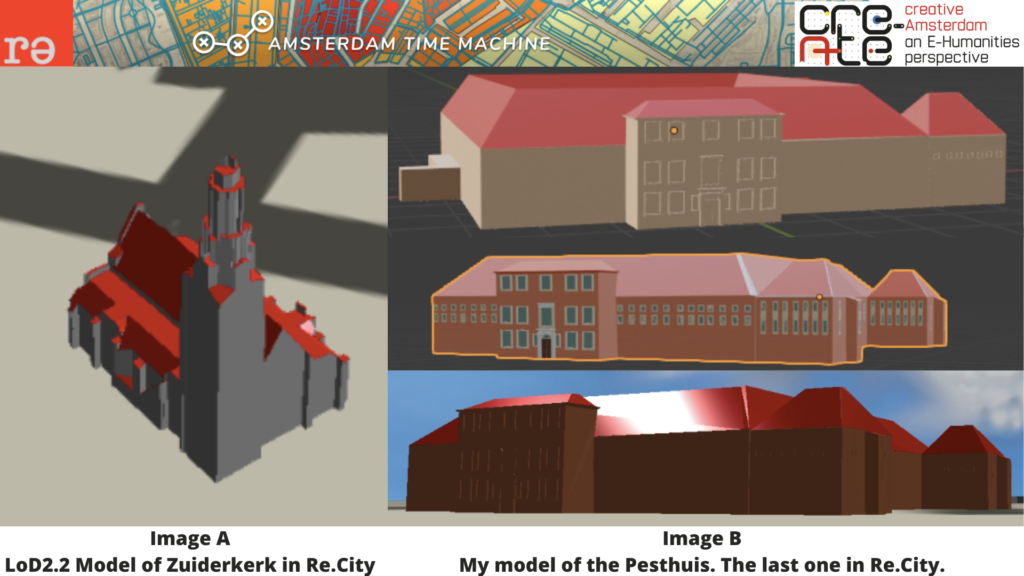
It is also possible to upload more complex 3D models individually. By associating a model to its Re.City building ID, the building should appear on the proper location in the 3D map. I used some buildings from the recently updated 3D BAG LoD2.2 (Image A). I also added my model of the “Pesthuis”, which I made in Blender (Image B). I chose this particular building because of my interest in medical history. Moreover, it is a historical building, demolished in 1937, and therefore is not in the 3D BAG database. Yet, even by associating the correct building IDs to these models, precisely assigning them to their correct location on the map turned out to be challenging. This problem seems to relate to the coordinate system Re.City uses, but this is an aspect that would need to be investigated further.
For some buildings, I associated pictures from the Beeldbank. In theory, Re.City should automatically recognize the windows and doors and adjust the 3D meshes accordingly. Unfortunately, this feature does not function properly yet. It worked for the “Spinhuis” (see Image C) but only after Google did some tweaks.
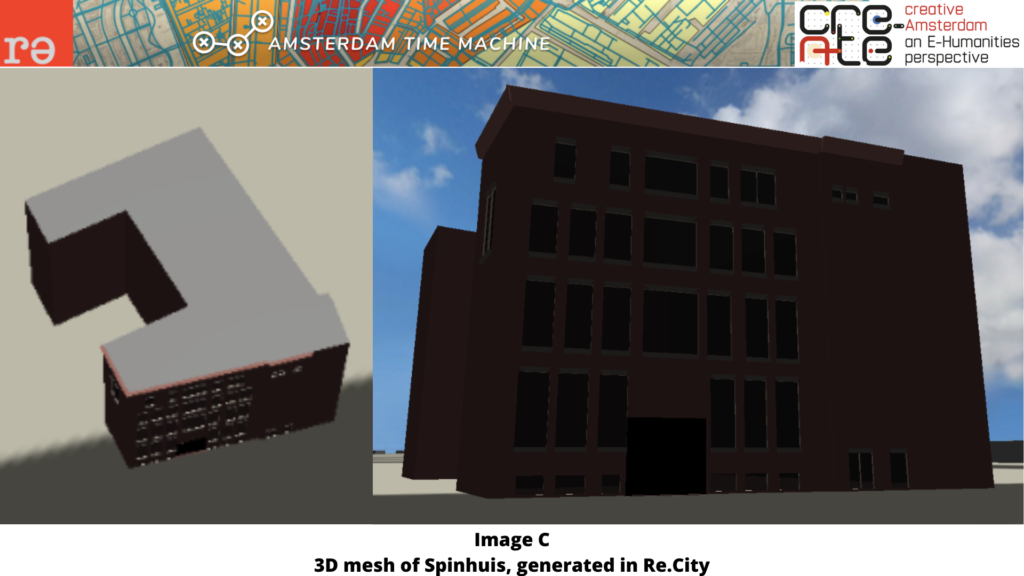
In conclusion, Re.City was more complicated to use than it seemed to be at the start of my internship. Fortunately, for many issues we encountered, the Google developer team was able to assist or provide a bug fix. So, the tool is undoubtedly promising, yet, some improvements need to be made to make it more effective and user-friendly for historical city reconstruction.
Daan Groot, intern at ATM
Explore the Re.City for yourself and read the blogpost of Google for more insight.